- Tableau Prep Conductor
- Tableau Prep Builder
- Tableau Prep Tutorial
- Tableau Prep Release
- Tableau Prep Builder
- Tableau Prep Builder Version 2019.4.2 and later and on the web: In the Profile pane, select the fields that you want to pivot, then right-click or Ctrl-click (MacOS) and select Pivot Columns to Rows from the menu. If using this option, skip to step 7. All versions: Click the plus icon, and select Add Pivot from the context menu.
- Tableau Prep is new tool from Tableau which helps to help people transform, clean and massage their data for analysis quickly. With simple drag and drop features to simplify the complicated tasks of joins, unions, pivots, aggregate or create calculate fields the data quickly.
Tableau Prep is a visual data preparation tool that allows you to get to data analysis faster by enabling the quick cleaning, reshaping, and prepping of data—using the same drag-and-drop paradigm as Tableau Desktop. Following the four month training period in Tableau and Alteryx products, Jack has been supporting a range of clients with their reporting and ETL pipelines. Jack is a keen Tableau Prep user and set up this User Group back in February 2020 as a means of bringing user stories together and watching the development of the tool.
Note: Starting in version 2020.4.1, you can now create and edit flows in Tableau Server and Tableau Online. The content in this topic applies to all platforms, unless specifically noted. For more information about authoring flows on the web, see Tableau Prep on the Web.
Sometimes analyzing data from a spreadsheet or crosstab format can be difficult in Tableau. Tableau prefers data to be 'tall' instead of 'wide', which means that you often have to pivot your data from columns to rows so that Tableau can evaluate it properly.
However you may also have scenarios where your data tables are tall and narrow and are too normalized to properly analyze. For example a sales department that tracks advertising spend in two columns, one called Advertising that contains rows for radio, television and print and one column for total spent. In this type of scenario, to analyze this data as separate measures you would need to pivot that row data to columns.
But what about pivoting larger data sets or data that changes frequently over time? You can use a wildcard pattern match to search for fields that match the pattern and automatically pivot the data.
Use one of the following options when pivoting your data:
Pivot columns to rows
Use wildcard search to instantly pivot fields based on a pattern match (Tableau Prep Builder version 2019.1.1 and later and on the web).
- Pivot rows to columns (Tableau Prep Builder version 2019.1.1 and later and on the web).
No matter how you pivot your fields, you can interact directly with the results and perform any additional cleaning operations to get your data looking just the way you want it. You can also use Tableau Prep's smart default naming feature to automatically rename your pivoted fields and values.
Pivot columns to rows
Use this pivot option to go from wide data to tall data. Pivot from columns to rows on one or more groups of fields. Select the fields that you want to work with and pivot the data from columns to rows.
Connect to your data source.
Drag the table that you want to pivot to the Flow pane.
Do one of the following:
- Tableau Prep Builder Version 2019.4.2 and later and on the web: In the Profile pane, select the fields that you want to pivot, then right-click or Ctrl-click (MacOS) and select Pivot Columns to Rows from the menu. If using this option, skip to step 7.
All versions: Click the plus icon, and select Add Pivot from the context menu.
Select Fields (Tableau Prep Builder version 2019.4.2 and later and on the web) Flow Step Menu (all versions)
(Optional) In the Fields pane, enter a value in the Search field to search the field list for fields to pivot.
- (Optional) Select the Automatically rename pivoted fields and values check box to enable Tableau Prep to rename the new pivoted fields using common values in the data. If no common values are found, the default name is used.
Select one or more fields from the left pane, and drag them to the Pivot1 Values column in the Pivoted Fields pane.
(Optional) In the Pivoted Fields pane, click the plus icon to add more columns to pivot on, then repeat the previous step to select more fields to pivot. Your results appear immediately in both the Pivot Results pane and the data grid.
Note: You must select the same number of fields that you selected in Step 5. For example if you selected 3 fields to initially pivot on, then each subsequent column that you pivot on must also contain 3 fields.
If you didn't enable the default naming option or if Tableau Prep couldn't automatically detect a name, edit the names of the fields. You can also edit the names of the original fields in this pane to best describe the data.
(Optional) Rename the new Pivot step to keep track of your changes. For example 'Pivot months'.
To refresh your pivot data when data changes, run your flow. If new fields are added to your data source that need to be added to the pivot, manually add them to the pivot.
Example: Pivoting on multiple fields
This example shows a spreadsheet for pharmaceutical sales, taxes and totals by month and year.
By pivoting the data you can create rows for each month and year and individual columns for sales, taxes and totals so that Tableau can more easily interpret this data for analysis.
Watch 'pivot on multiple field' in action.
Use wildcard search to pivot
If you work with large data sets or if your data frequently changes over time, starting in Tableau Prep Builder version 2019.1.1 and on the web, you can use a wildcard search when pivoting columns to rows to instantly pivot your data based on a wildcard pattern match.
If new fields are added or removed that match the pattern, Tableau Prep detects the schema change when the flow is run and the pivot results are automatically updated.
Connect to your data source.
Drag the table that you want to pivot to the Flow pane.
Click the plus icon, and select Add Pivot from the context menu.
In the Pivoted Fields pane, click on the link Use wildcard search to pivot .
Enter a value or partial value that you want to search for. For example, enter Sales_ to match fields that are labeled as sales_2017, sales_2018 and sales_2019.
Do not use asterisks to match the pattern unless they are part of the field value that you are searching for. Instead click the Search Options button to select how you want to match the value. Then press Enter to apply the search and pivot the matching values.
(Optional) In the Pivoted Fields pane, click the plus icon to add more columns to pivot on, then repeat the previous step to select more fields to pivot.
If you didn't enable the default naming option or if Tableau Prep couldn't automatically detect a name, edit the names of the fields.
To refresh your pivot data when data changes, run your flow. Any new fields added to your data source that match the wildcard pattern are automatically detected and added to the pivot.
If the results aren't what you expect, try one of the following options:
Enter a different value pattern in the Search field and press enter. The pivot will automatically refresh and show the new results.
Manually drag additional fields to the Pivot1 Values column in the Pivoted Fields pane. You can also remove fields that were added manually by dragging them off the Pivot1 Values column and dropping them in the Fields pane.
Note: Fields that were added from the wildcard search results can't be removed by dragging them off the Pivot1 Values column. Instead try using a more specific pattern to match the search results you are looking for.
Pivot rows to columns
In Tableau Prep Builder version 2019.1.1 and later and on the web, pivot rows to columns if your data is too normalized and you need to create new columns - going from tall data to wider data.
For example if you have advertising costs for each month that includes all advertising types in one column, if you pivot the data from rows to columns you can then have a separate column for each advertising type instead, making the data easier to analyze.
You can select one field to pivot on. The field values for that field are then used to create the new columns. Then, select a field to use to populate the new columns. These field values are aggregated and you can select the type of aggregation to apply.
Because aggregation is applied, pivoting columns back to rows won't reverse this pivot action. To reverse a row to column pivot type, you will need to undo the action. Either click the Undo button on the top menu, remove the fields from the Pivoted Fields pane or delete the pivot step.
Connect to your data source.
Drag the table that you want to pivot to the Flow pane.
Click the plus icon, and select Add Pivot from the context menu.
In the Pivoted Fields pane, select Rows to Columns from the drop-down list.
- (Optional) In the Fields pane, enter a value in the Search field to search the field list for fields to pivot
Select a field from the left pane, and drag it to the Field that will pivot rows to columns section in the Pivoted Fields pane.
Note: If the field you want to pivot on has a data type of date or datetime, you will need to change the data type to string to pivot it.
The values in this field will be used to create and name the new columns. You can change the column names in the Pivot Results pane later.
Select a field from the left pane and drag it to the Field to aggregate for new columns section in the Pivoted Fields pane. The values in this field are used to populate the new columns created from the previous step.
A default aggregation type is assigned to the field. Click the aggregation type to change it.
In the Pivot Results pane, review the results and apply any cleaning operations to the new columns that you created.
If the field being pivoted has a change in its row data, right-click or Ctrl-click (MacOS) on the Pivot step in the flow pane and select Refresh.
Tableau Prep Builder recently released an update (2019.3) that included a brand-new tool: The Script Step. This step lets us run an R or Python Script upon data in a flow, which opens our analysis up to the vast range of functions available in both programs. Seriously, the possibilities are endless!
In this article we will focus on running an R Script, as:
- a) there aren’t many R-specific guides out there, and
- b) R has a fairly intuitive scripting language and is easier to get up-and-running than Python (in my opinion).
Why should I bother?
Whilst Tableau Prep can do a lot of great things, I believe it is missing some essential steps, namely:
- Indexing
- Sorting
- Flagging outliers
- Ranking*
*Tableau Prep version 2020.1 now allows users to create Level of Detail Calculations, including Rank, so you will only need to use an R script to Rank if using an earlier version
I would also include Top N filtering on this list, but we can do this with two steps: Ranking (via R script) and Filtering (via Tableau Prep).
There is also the huge range of data science functionality that R offers (clustering, modelling, machine learning…) but we’ll leave that for a future article!
Before we get started
- 1. Download & Install the latest version of R from here
- 2. Download & Install Tableau Prep 2019.3 (or above) from here
You can also download the materials & examples used in this article (including the packaged flow file) here.
How it works
Tableau Prep runs your R Script via the Rserve Server. This means that you need to install and run the Rserve package within R Console before you can run your script.
To install and run Rserve:
- 1. Open R Console
If using Rserve for the first time:
- 2. Type in the following code into R and press Enter:
install.packages(“Rserve”)
- 3. At this point you will be asked to select a CRAN mirror for use in this session – select one in the country closest to you (here we used UK (London 1) [https]).
If Rserve is already installed:
- 4. Type in the following code into R and press Enter:
library(Rserve)
Rserve()
You should now have the Rserve Server up and running. Your R workspace should look as follows:
Setting up the Script step in Prep
1. Add a Script step to your data
- 2. Make sure the connection type is Rserve
- 3. Select “Connect to Rserve Server” input the following credentials, and click Sign In.
Server: localhost
Port: 6311
Note: Port 6311 is the default port for plaintext Rserve servers. If your server is SSL-encrypted you will need Port 4912 and you may need to enter a username and password.
You are now ready to upload an R script and apply it to your data.
How an R Script works
R Scripts are lines of code that perform certain tasks within R. They can be saved and then referenced later, removing the need to write new lines of code every time.
You will need to write and save your R code separately to Tableau Prep as a *.R file, then upload it via the script step.
There are some important things to know about the format of an R script.
- Your input and output will always be in the form of a data frame. The table of data that flows into the script step in your prep flow forms the data frame (called “df”) by R.
- You need to reference specific column names. You cannot design an R script that dynamically takes the first column in your data, for example.
- The columns being referenced in the R script cannot have spaces, special characters (e.g. % or £) or begin with a number, so you may need to rename columns before the script tool. This is because R will interpret these characters as a command.
- You must list all columns that you want to output in your data frame. Any missing columns will not be returned in the output of the Script step.
- For this reason, it may be easier to only apply a script to the columns you need to manipulate and then join these back onto the rest of the data afterwards.
- Your script should contain a function that does something specific to your data. All manipulation is done via an R function. You can explore R functions in R’s official documentation here.
- You will need to specify the data types of your outputs. This means including an additional function called getOutputSchema.
Creating an R Script
- 1. Within R Console, choose File > New Script
2. Once you have written your script, save your file via File > Save
This is the file you will upload to Tableau Prep in the File Name section (you will also need to specify a function).
Layout of an R Script in Tableau Prep
The generic format of an R script that will work in Tableau Prep is as follows:
Practical Examples:
The examples below will use the following simple table of data. Feel free to copy it into Excel and connect to the file in Tableau Prep.
You can also download the materials & examples used in this article (including the data) here.
Note that all the example scripts can be easily customised to match your own data. Just change the names of the columns according to the generic layout given beforehand.
Example 1 – Indexing a Column
Aim: to produce a column of integers that label each record in our dataset from 1 to 12*.
* 12 is not a hard-coded number; the script will calculate how many rows are in the data.
Desired Output:
R Script:
Index_function <- function(df) {
Name <- df$Name
df$Age <- df$Age
df$Index <- 1:nrow(df)
return(df)
}
getOutputSchema <- function() {
return(data.frame(
Name = prep_string(),
Age = prep_int(),
Index = prep_int()
))
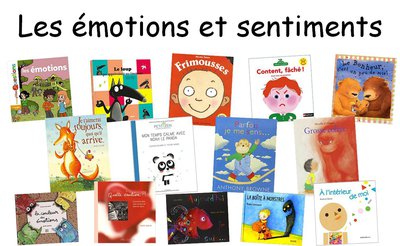
}
Note that the R Function 1:nrow(df) takes the entire dataframe, df, as an argument.
In Tableau Prep, upload the R script above and type “Index_function” (the name of the function) into the Function Name field. Press Enter to apply your script.
Example 2 – Sorting a Column
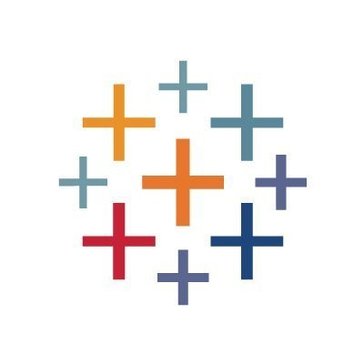
Aim: to sort the data by the value of a column, in this case Age in ascending order (smallest to largest).
Desired Output:
R Script:
Sort_Age <- function(df) {
Name <- df$Name
Age <- df$Age
df <- df[order(df$Age),]
return(df)
}
getOutputSchema <- function() {
return(data.frame(
Name = prep_string(),
Age = prep_int()
))
}
The command line df <- df[order(df$Age),] replaces the data frame with a new data frame in the correct order. Note that the R Function order takes the column df$Age as an argument, and sorts it in ascending order.
To reverse the order of the sorting (largest to smallest), simply replace df$Age with -df$Age
In Tableau Prep, upload the R script above and type “Index_function” (the name of the function) into the Function Name field. Press Enter to apply your script.
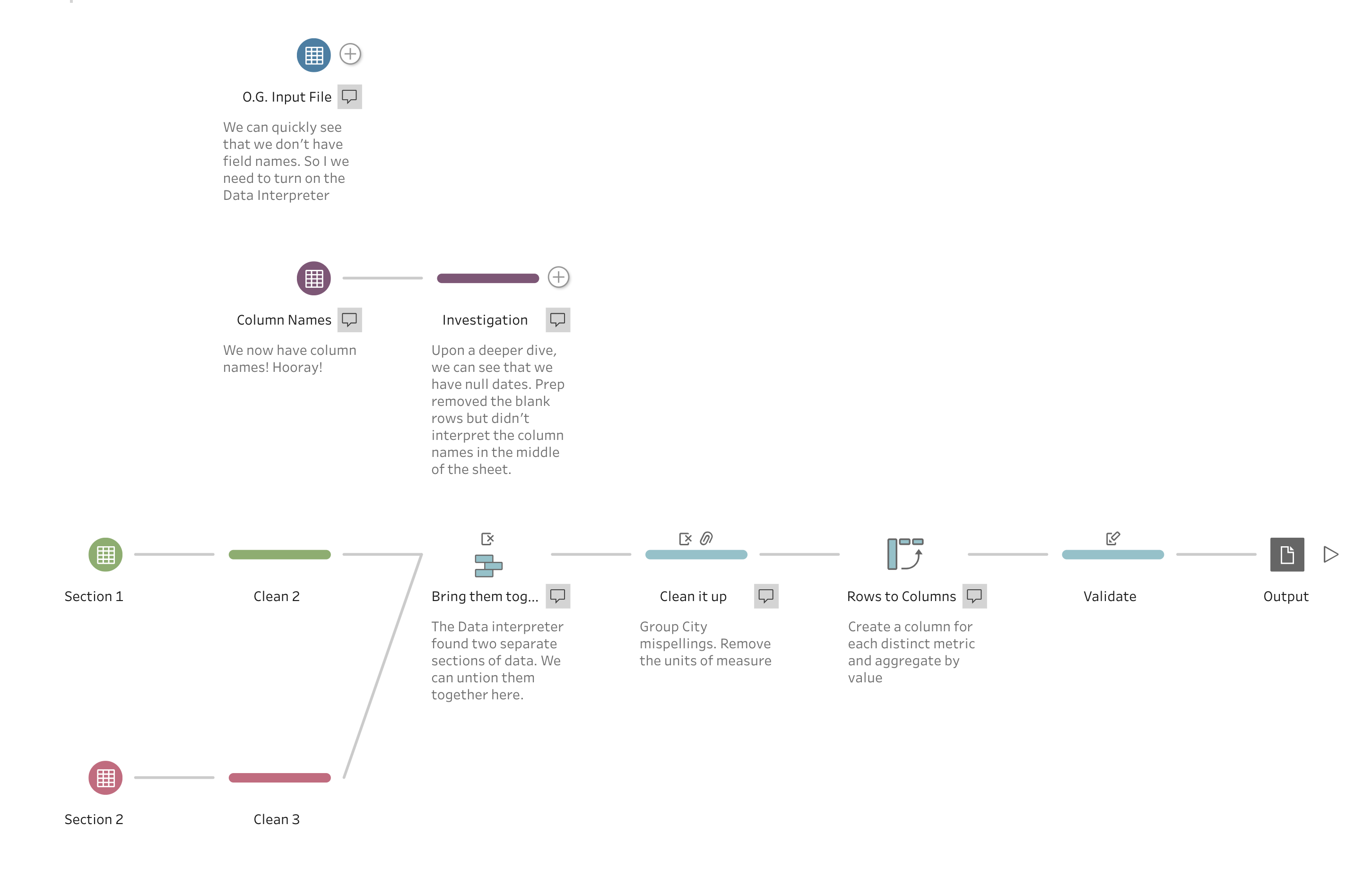
NOTE: You can also divide your data into groups and sort by group – e.g. split by gender and then sort by age. To do this you would include the gender column as the first argument of the order function:
df <- df[order(df$Gender, df$Age),]
Example 3– Creating an “Outlier” Flag
Aim: to produce a flag for when an Age is an outlier, defined as a data point above Q3 + 1.5 times the IQR. Here 1 = outlier and 0 = not outlier.
Desired Output:
R Script:
Outlier <- function(df) {
Name <- df$Name
Age <- df$Age
df$Outlier_Flag <- ifelse(test = Age > quantile(Age, probs = 0.75) + IQR(Age) * 1.5,
yes = 1,
no = 0)
return(df)
}
getOutputSchema <- function() {
return(data.frame(
Name = prep_string(),
Age = prep_int(),
Outlier_Flag = prep_int()
))
}
Note that the R Function ifelse takes the column Age as an argument, and also references other functions inside it.
In Tableau Prep, upload the R script above and type “Index_function” (the name of the function) into the Function Name field. Press Enter to apply your script.
Tableau Prep Conductor
Example 4 – Ranking a Column

*Tableau Prep version 2020.1 now allows users to create Level of Detail Calculations, including Rank, so you will only need to use an R script to Rank if using an earlier version
Aim: to produce a column of integers that rank each value in the ‘Age’ column from 1 (highest age) to 12 (lowest age).
Desired Output:
R Script:
Tableau Prep Builder
Rank_Age <- function(df) {
Name <- df$Name
Age <- df$Age
df$Rank_of_Age <- (nrow(df)+1) – rank(Age , ties.method= “max”)
return(df)
}
getOutputSchema <- function() {
return(data.frame(
Name = prep_string(),
Age = prep_int(),
Rank_of_Age = prep_int()
))
}
Note that the R Function rank(Age , ties.method= “max”) takes one column, Age, as an argument.
NOTE: This ranking method uses the Competition (1,2,2,4) method. For other options, look into the ties.method options within the rank() function.
We could reverse the order of the Rank by replacing (nrow(df)+1)- rank(Age, ties.method= “max”) with rank(Age, ties.method= “min”). Again, this would use the Competition (10,9,9,7) ranking method.
In Tableau Prep, upload the R script above and type “Rank_Age” (the name of the function) into the Function Name field. Press Enter to apply your script.
You can also download the materials & examples used in this article (including the packaged flow file) here.
Testing your Script in R
I find it helpful to test the script in R before attempting it within a script tool. Some tips here:
- Output your data as a *.csv file from Tableau Prep at the point before you will use a script tool
- Upload this file to R using the read.csv tool.
Ages_Data <- read.csv(“[file path & file name].csv”)
- Turn this into a data frame called “df” by using the data.frame function.
df <- data.frame(Ages_Data) - Check the column names of your data frame using the names function. If they do not match your data then use the second line of this script.
NOTE: Command lines beginning with # are comments and do not affect the script
# To check column names
names(df)
Tableau Prep Tutorial
# To change column names
names(df) <- c(“Name”, “Age”)
- Highlight the first half of your function (everything above getOutputSchema) and press CTRL+R. This will run your function.
- Test your function
Rank_Age(df)
What next?
I spoke at the Tableau Prep Virtual User Group on Thursday 30th April 2020, showing users how you can expand the current capabilities of Tableau Prep by leveraging R Scripts, including how to sort, rank, index your data, flag outliers, and more. Catch up on the video here
Tableau Prep Release
I believe the community is only just beginning to scratch the surface of the possibilities within the Script tool. Look out for future articles on data science functionality & advanced analytics, and please help spread the word by sharing this article!
Tableau Prep Builder
How to use R Script with Prep Builder is also covered in more detail in our 2-day Prep Builder training course, which touches on all aspects of Prep functionality. Please contact uswith any enquiries and booking requests, we would be happy to accommodate you.
